In the previous blog, Padmashree Pratap explained how Matillion’s Shared Jobs can transform ETL workflows by enhancing efficiency, reducing maintenance, and promoting better collaboration. The benefits of Shared Jobs, such as their reusability across projects, streamlined updates, and improved performance, were thoroughly discussed. Building on those insights, this blog explores real-world examples of how Shared Jobs are implemented to tackle specific challenges and optimize ETL processes. By examining these practical applications, readers can see how the concepts from the previous blog are applied in various scenarios, highlighting the benefits of adopting Shared Jobs .
Example 1 – Broadcasting Custom Stats
Requirement:
To ensure updates on key measures are provided after every data refresh (ETL run), a company needs a streamlined and consistent process for calculating and broadcasting custom statistics across various workflows. This is especially important for maintaining accurate and up-to-date reporting across different departments or regions.
Challenge:
Without using shared jobs, each ETL workflow would need to calculate and update key performance indicators (KPIs) independently. This could lead to inconsistent metrics due to variations in calculation methods, increased manual effort to maintain and update multiple scripts, a higher likelihood of errors and discrepancies in reports, and difficulties in synchronizing data insights across departments. Additionally, scaling such a fragmented process across multiple regions or departments becomes inefficient, potentially leading to delays in decision-making.
Solution:
A multinational logistics corporation specializing in accurate reporting of sales metrics across different regions implements Matillion ETL’s shared job functionality to streamline this process. The shared job, depicted in the screenshot below, serves as a centralized engine for calculating and broadcasting custom statistics derived from various operational data sources. This job can be called from an orchestration job and generated into a shared job, allowing flexibility in configuring inputs, such as the sales table, and defining the output destination for statistical results using job variables.
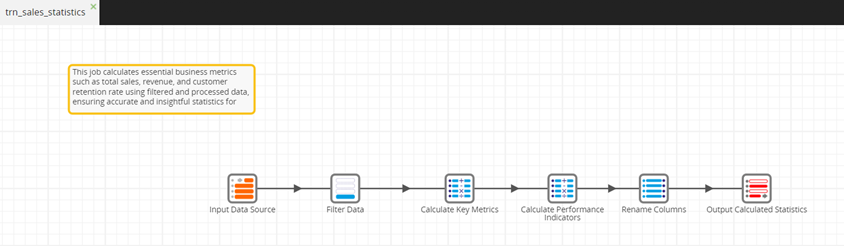
After the sales teams in both the US and the UK complete their quarterly sales data extraction, they trigger the shared job. This instantly updates the sales performance KPIs visible to all relevant stakeholders, ensuring that both the US and UK departments are synchronized with the latest data insights, maintaining uniformity and accuracy in their reports. The orchestration job of the US sales team, depicted in the screenshot below, demonstrates the process of triggering the shared job after data extraction.
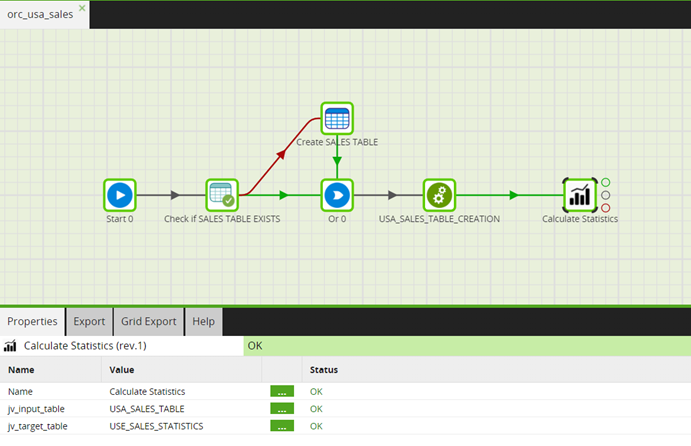
Similarly, the orchestration job of the UK sales team, shown in the following screenshot, shows the same process of triggering the shared job post data extraction.
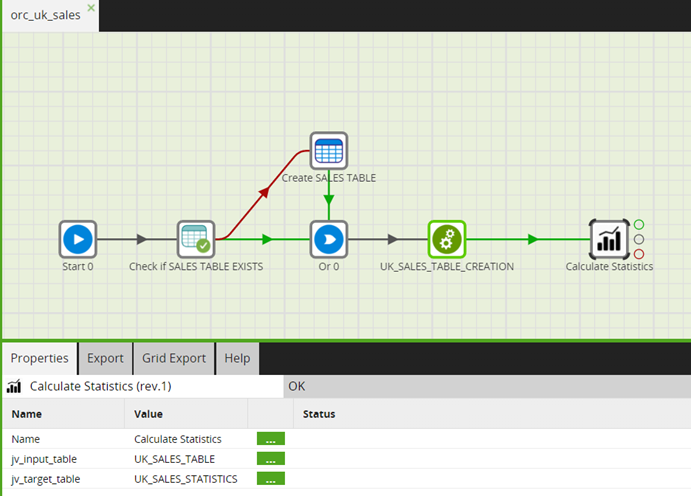
This approach is particularly useful for companies with multiple markets in various countries, as it automates KPI dissemination, reduces manual effort, guarantees consistent data processing, adapts easily to changes, and fosters collaboration through reliable, comparable data. By utilizing shared jobs, the company ensures uniform and accurate reporting, facilitating strategic decision-making with consistent and reliable data.
Example 2: Centralized Data Validation
Envision a healthcare provider that needs to authenticate patient data from different sources before importing it into their central database. A shared job is designed for data validation, which is then employed across all ETL workflows dealing with patient data. This approach guarantees that all data follows the same validation rules, enhancing data quality and reducing redundancy in job definitions.
In this case, the shared job could include checks for data completeness, format validation, and business rule enforcement. By centralizing these checks in a shared job, the healthcare provider ensures that all incoming data meets the same high standards, reducing the risk of errors and improving the reliability of their database.
Common User Scenarios:
- Patient Registration System Integration:
When a new patient registers through various systems (e.g., online portal, in-clinic registration, or mobile app), the Data Validation Shared Job ensures that all collected data is complete and formatted correctly before it is stored in the central database. This prevents issues such as missing contact information or incorrect date formats. - Medical Records Synchronization:
As patient records are updated from different departments (e.g., laboratory results, radiology reports, or prescriptions), the Data Validation Shared Job verifies that all entries comply with predefined standards. This ensures that critical patient information is accurate and up to date across all systems. - Compliance and Reporting:
Regulatory compliance often requires rigorous data validation. The Data Validation Shared Job enforces compliance with healthcare regulations such as GDPR (General Data Protection Regulation), ensuring that all patient data adheres to legal and ethical standards. This simplifies the process of generating compliant reports for regulatory bodies.~ - Billing and Claims Accuracy:
In healthcare organizations, billing and claims accuracy is crucial to ensure proper reimbursement and financial integrity. The Data Validation Shared Job can be instrumental in validating billing and claims data before submission to insurance companies or government agencies. By centralizing these validation checks, healthcare providers can minimize billing errors, reduce claim rejections, and improve overall revenue cycle management. By incorporating these scenarios into the Data Validation Shared Job, the healthcare provider can handle diverse data validation needs efficiently and consistently, enhancing overall data quality and reliability.
Example 3: Streamlining Data Ingestion
Requirement:
A multinational corporation operating across various regions needs to automate the ingestion of daily sales transactions from diverse sources including POS terminals, online platforms, and regional warehouses. This automation is essential for consolidating data into a unified data warehouse, enabling comprehensive analytics across product categories and geographical locations.
Challenge:
Without leveraging shared jobs in Matillion ETL, the corporation would face significant challenges. Each data extraction, transformation, and loading process would need separate configuration and management. This manual approach increases the risk of errors and inconsistencies in handling data from diverse platforms. Additionally, without shared workflows, it would be harder to grow operations efficiently. Managing large amounts of data from multiple systems and locations could become inefficient and challenging. The corporation may struggle to maintain data accuracy and obtain timely insights essential for comprehensive sales performance analysis and operational decision-making.
Solution:
Matillion ETL automates the extraction, transformation, and loading processes, ensuring seamless integration of data from various sources such as databases, APIs, and cloud storage into a centralized data warehouse. The screenshot below illustrates a job in Matillion ETL that invokes a shared job, demonstrating its capability to flexibly configure through job variables. These variables empower users to specify data sources for ingestion and configure connection details, enabling customization based on criteria such as source system. This flexible setup facilitates automation and streamlines data ingestion processes for organizations.
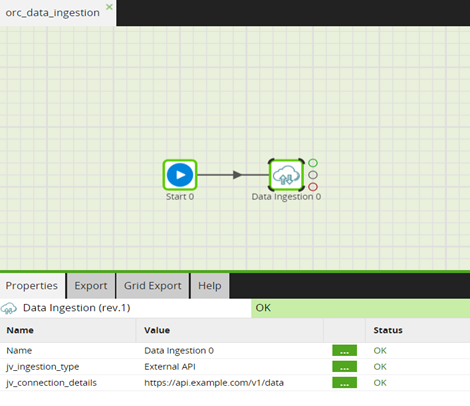
The screenshot below provides a glimpse of how the ingestion job can be built using Matillion ETL, showcasing its capability to create reliable data ingestion workflows integrated with powerful data transformation capabilities. Utilizing Matillion’s IF component, organizations can dynamically route data ingestion processes based on conditions such as data source type or ingestion requirements. Following ingestion, Matillion enables efficient data transformation using its comprehensive suite of transformation tools.
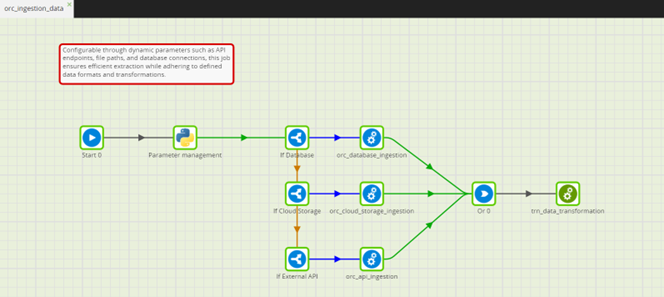
By utilizing Matillion ETL’s flexible job building features for data ingestion and transformation, supermarket chains and other retail enterprises can streamline operations and seamlessly integrate a variety of data sources.
Matillion’s shared jobs provide a considerable edge over normal jobs, particularly when managing reusable ETL components across several projects. They enhance maintainability, promise consistency, enable efficient parallel processing, foster collaboration, streamline testing and debugging, and offer cost efficiency. By harnessing shared jobs, organizations can elegantly streamline their ETL processes, cut down maintenance overhead, and improve data quality. They help ensure that best practices are consistently applied, reduce the risk of errors, and make it easier to scale your data processing capabilities.
Ready to streamline your ETL processes? Start exploring Matillion’s shared jobs today and see the difference.
References
- Matillion. (2018, September 28). Error Handling Options in Matillion ETL – Creating a Shared Job. https://www.matillion.com/resources/blog/error-handling-options-in-matillion-etl-creating-a-shared-job
- Matillion. (2020, July 13). Import and Export Shared Jobs with Matillion ETL. https://www.matillion.com/resources/blog/import-and-export-shared-jobs-with-matillion-etl
- Matillion. (n.d.). Shared Jobs – Matillion Docs. https://docs.matillion.com/metl/docs/3070195/shared_jobs