In today’s fast-paced digital landscape, organisations are increasingly relying on modern cloud-native platforms like Google BigQuery, Azure Fabric, Snowflake, and Databricks to power their data-driven decision-making. These platforms offer scalability, flexibility, and advanced analytics capabilities that are crucial for staying competitive. Yet, SAP seems stuck in the past, stubbornly refusing to acknowledge this multi-platform reality. I bet even SAP does not run all its analytics from a single (SAP) platform (and I would love to see the evidence to prove that ?). By denying customers seamless ways to export data to these platforms, SAP’s approach is creating significant challenges for its users and driving them towards third-party solutions.
SAP’s outdated approach to data integration
SAP’s integration tools are ill-suited for the needs of the modern data stack. Let’s have a look at the options:
– SAP Landscape Transformation Replication Server (SLT)
As a trigger-based replication server, it is theoretically a suitable candidate for integration. However, it is mainly geared towards SAP-to-SAP replication and, outside of SAP, it can only land data in legacy databases like DB2, SQL Server, or Oracle. None of these can be classified as ‘modern data platforms’.
– SAP Data Services (BODS, BODI)
SAP Data Services is built on a 30(!)-year-old client/server architecture, unsuitable for cloud environments, and lacks meaningful data streaming capabilities.
– SAP DataSphere Premium Outbound
SAP DataSphere Premium Outbound, while technically capable of provisioning data, is far too complex and expensive to be practical if used solely for data export into a third-party cloud environment. And despite the label of ‘Premium’ the supported target destinations is still rather limited.
Limitations like this make SAP’s own solutions impractical for organisations that need quick and reliable ways to integrate their SAP data with cutting-edge cloud ecosystems.
Customers are turning to third-party alternatives
For me as a consultant, it is frustrating to see customers wasting time trying to make data extraction from SAP work following SAP’s biased guidance. In recent months, I have seen a global enterprise struggling for months with SLT/Data Services and not getting anywhere. Another global enterprise has decided to abandon their SAP DataSphere project after nearly two years and underwhelming results. SAP claims to put the customer first, but that only seems to be true if the customer is willing to exclusively use SAP’s products.
Faced with SAP’s shortcomings, many organisations are looking elsewhere. Third-party tools like Fivetran, SNP Glue, Theobald and Qlik Replicate are becoming the go-to options for extracting SAP data and moving it to modern cloud platforms. These solutions are not only more user-friendly and efficient but also offer robust support for a wide range of cloud-native systems.
What these tools have in common is their ability to do what SAP’s own offerings cannot: make it simple and seamless to integrate SAP data with platforms like BigQuery, Snowflake, or Databricks.
At the right of this text is a table with some great 3rd party tools which all blow SAP out of the water when it comes to replicating data to a cloud data platform (there is little evidence published, but I am happy to stand by this claim as I have seen it many times). If you are looking for guidance on which tool is best for your organisation please get in touch as Snap Analytics’ methodology for this is efficient and proven successful. It looks at your complete landscape for analytics (source- and target destinations), the impact on your SAP ERP workload, your in-house skills, costs and other factors).
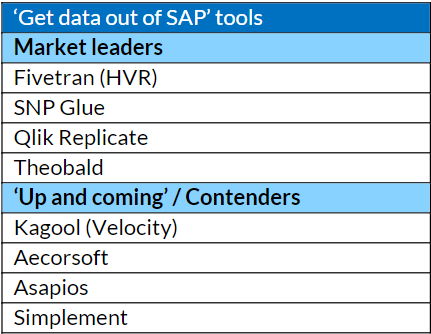
The impact on customers
SAP’s refusal to adapt to the needs of the modern data landscape is hurting its customers. Organisations that rely on SAP for their core business processes are being forced to choose between two suboptimal options:
- Stick with SAP’s in-house tools: This often results in higher costs, increased complexity, and slower time-to-value.
- Invest in third-party solutions: While these tools are effective, they add additional vendors, integration layers, and costs to an already complex IT landscape.
In either case, customers bear the burden of SAP’s unwillingness to acknowledge the reality of multi-cloud and hybrid environments. The inefficiencies created by SAP’s closed-off approach can delay data-driven decision-making, inflate expenses, and limit the ability to leverage advanced analytics capabilities.
Why SAP needs to change
The reality is clear: the enterprise data landscape is no longer confined to monolithic systems or single-vendor ecosystems. Organisations are building diverse, cloud-centric data architectures. SAP should recognise this, and put the customer first again. This means embracing openness and interoperability, rather than continuing to push inferior, SAP-centric solutions.
By providing robust, user-friendly mechanisms for exporting data to non-SAP platforms, SAP could reclaim the trust of its customers and cement its place as a leader in enterprise data management. Customers are happy to pay for a premium service to get data out of SAP ERP and into the cloud – as long as that service is truly premium in the technical sense, not just in name and price.
Until then, customers will seek a better solution from a 3rd party provider.