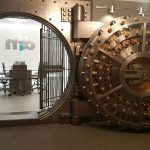
Part 1 – What is Data Vault?
August 3, 2020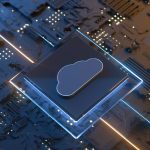
The future of SAP Business Warehouse
February 21, 2021If you’re wondering what exactly Data Vault is then check out Part 1 of our Data Vault blog where we outline exactly what Data Vault is, otherwise keep reading to find out all the great benefits of using Data Vault methodology!
Scalability
Probably the biggest benefit given the current data landscape that we find ourselves in is the scalability of data vault. From my own experiences, data warehouses over time become more resistant to change and much less flexible to adapt to the changing needs of the business. Once certain reports and datasets have been created, in order to develop new functionality all of the existing functionality will need to be tested to ensure that it hasn’t broken, and refactored if it is. This is where Data Vault really helps as it is easily extensible without the worry about losing any historical data. New link tables and satellites can easily be added to the model, and similarly other link tables can be closed off when required. When virtual information marts are used this means it’s even more adaptable as it’s only the joins that will require updates to handle the changes.
Flexibility
Link tables mean that relationships are modelled in a way that it assumes that there will be many-to-many requiring virtually no work when a relationship changes. All historical data is tracked by default in the Data Vault by using the satellite tables meaning again the flexibility is there to report on the historical changes of the data warehouse. For example, if the business decides that actually they need to report on the historical status of a master data object that previously wasn’t a requirement, this can simply be provided by altering the joins to pull the correct data from the master data satellite tables. Previously we would have probably thought that using this approach would have caused lots of redundant data to be stored, however with the rise of cloud storage the costs for storing are so trivial that this now means we can design and build with future change in mind.
Auditability and Traceability
This tracking of history also means that the data warehouse is fully auditable. Data Vault practitioners like to say that it becomes the “single version of the facts” rather than the “single version of the truth”. All data is loaded into the data vault exactly as it was in source, rather than just cleansed data for a specific reporting purpose. This means that you can fully reconcile the data back to the source system at the point of entry, and it also becomes a lot easier to track the data lineage. Business users will no longer see the data warehouse as a ‘black box’, but instead it will become the trusted source for all of their business data!
Big Data & Loading
Traditional modelling approaches were created long before the advent of semi-structured and machine generated data. In Data Vault there isn’t the requirement to cleanse and conform the data to ensure star schema compliance which means that huge volumes of data can be loaded very quickly. Data Vault also uses hashed keys meaning that there is reduced dependencies during loading between hub, satellite and link tables meaning that they can be loaded in parallel, making use of scalable processing power that is available with cloud data warehouse solutions like Snowflake. Data vault also uses the concept of a virtual information mart layer on top of the data vault for reporting which again means increased performance as cubes don’t need to be persisted and loaded. This is a key feature that helps to ensure that real-time data warehousing can be realised.
All of this sounds great, so why isn’t data vault more widely used when building out data warehouses? I think the simply answer can be put down to a couple of key factors. The first is that cloud data warehousing has only recently started to be adopted, and it’s only with the scalability of cloud storage and processing that means that a data vault structure is truly viable. The other key factor is that if a project that is being delivered is a small project then it may be that building it using a data vault methodology is overkill. However, given the increasing importance of data and stricter data regulations (e.g. GDPR) I would argue that in many cases Data Vault methodology would be the right choice to ensure a modern and scalable data warehouse.
Written by Tom Bruce the Delivery Lead and Co-founder of Snap Analytics.