Modern Data Warehouse – Snowflake & Fivetran
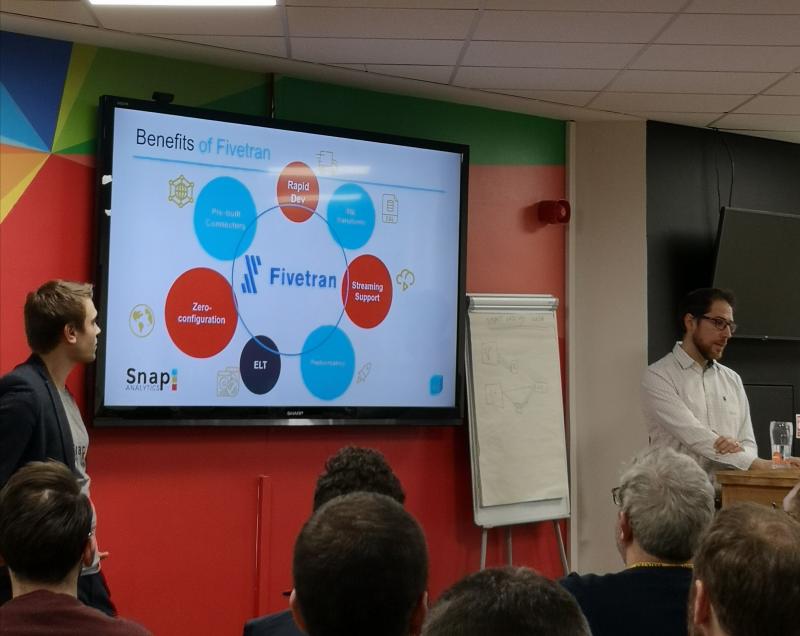
Picture this: you’ve decided to embark on an analytics project and find yourself in the offices of an experienced analytics team. To the untrained eye it might look like a bunch of people in comic book t-shirts staring into seemingly infinite streams of mysterious numbers, symbols and letters.
What are they all doing? You might well ask! We’re the first to admit that data analytics is hardly the most accessible business. With that in mind, here is a handy cut out and keep guide to the different types of people you want to work on your data.
Business analyst, aka the Go-between
One of your main points of contact on any project, the first thing you’ll notice about the business analyst is that they speak a language you understand. This is because the role isn’t explicitly technical, in fact some people believe it’s better for them to be focussed solely on business needs. It’s still really important that they do have a good working knowledge of the data so they can understand and represent all parties and be able to explain in comprehensible terms the possibilities and limitations of what we can deliver.
Solution architect, aka the Overseer
Like a bricks and mortar architect, this person is responsible for producing the blueprint for your project and setting out a workable solution from end-to-end. To expand the building analogy, they also take on some of the duties of a site manager, deciding on the tools we will need to use, such as AI or machine learning. The solution architect is the lynchpin, connecting everyone involved in the project and providing you with an overview.
Data modeller, aka the Translator
This person translates different raw data sets into accessible formats so that it can be usefully queried in order to provide the answers your business needs. Data modellers use the context and knowledge of the business provided by the Business Analyst to design how the data is stored, making complex data accessible and understandable for end users in the business.
Data engineer, aka the Wrangler
Every team needs someone who’s good at bringing things together. The data engineer (once known as the ETL developer) extracts the data from several often complex systems and wrangles it, filters it and loads it into a user friendly and accessible data model – designed by the data modeller – that your business users and data scientists will understand. The data engineer needs great communication skills as they have to understand what it is your business wants to get out of the finished database and ensure that the solution provided meets those expectations.
Data storyteller, aka the Visionary
Previously known as a data analyst, a bit of a dry moniker for someone who can visualise data and produce reports that are easily understood by people with little knowledge of analytics. A good storyteller turns data analyses into meaningful, understandable and actionable insights that will benefit your business.
Data scientist, aka the Prophet
So, data analytics has provided the initial actionable insights for your business, but what if you want more? The data scientist is your new best friend, looking even deeper into the figures for untapped findings, identifying new correlations and making your data work harder.
Finally, let’s not forget the invaluable input of the project managers who keep the whole thing on the road and the vital role of the experts within your business. At Snap Analytics we take an integrated approach, connecting our expertise across the different data roles with your business experts. Working closely together we will help you to gain new insights from your data in order to make better and more informed decisions.
In 2013 Dan Ariely, professor of behavioural economics at Duke University, got the analytics world all aquiver when he stated: “Big data is like teenage sex: everyone talks about it, nobody really knows how to do it, everyone thinks everyone else is doing it, so everyone claims they are doing it.” His risqué comments appeared in the midst of a data revolution, the experience of which was less than satisfactory for many companies.
Six years on and we’re doing it better. However, AI is in danger of being in the same position as Big Data was six years ago. Some data strategists would have you believe that AI and its bedfellow machine learning are better than sex. But as with anything in the data revolution, don’t expect to achieve total fulfilment overnight.
Cost vs kudos
A report from the McKinsey Global Institute predicts that early adopters of this technology could grow the value of their business by 120%. It adds that those who fail to jump on board the AI gravy train could lose a fifth of their cash flow. No surprise that companies are throwing money at the problem – but not necessarily always for the right reasons.
Kudos is not enough of a reward for a business to pump considerable sums of money into an AI project. A survey by the US analytics company Figure Eight showed that the majority of companies were spending at the very least $50,000, rising to over $5 million for those serious about making it a central part of their business.
AI has now been around for two decades. Advances in tech plus heavy investment from the likes of Google mean that the cost of leveraging AI tools will continue to fall, levelling the playing field and allowing smaller businesses to utilise AI. If you’ve made it your business to amass a wealth of clean, properly managed data you are already well positioned to launch an effective AI project.
Let’s not get ahead of ourselves
With any data project, there are things you need to think about before you get started. If you’re looking to get started with AI specifically, first consider whether the problem you would like to address is best served by the technology. Don’t expect AI to act as a sort of panacea; you need to be deploying it in the right way and for the right reasons. If you’re unsure, talk to an expert first (yes, we can help with that) to assess what sort of data analysis would be best for your particular problem.
Maybe there is an area of your business you are certain would benefit from an AI solution – if only you could convince the CFO to invest. If they’re keeping a tight hold of the purse strings ask yourself: does this align with the greater corporate strategy? If no, you’d probably be better focusing your efforts somewhere that does.
Finally, when you have identified the right AI project and hired yourself a crack analytics specialist (hello), don’t assume that the thing will just run itself. AI is smart but it still needs help. That means putting together the right team – and not just a couple of people borrowed from the IT department. Successful AI needs buy-in from people who understand the business need and who are working with the numbers on a daily basis.
Get it right and you’ll transform your AI experience from a meaningless one night stand to a satisfying relationship that grows into something really special.